Introduction
I researched and furthered the development of a set-based approach for design space exploration, providing an alternative methodology for addressing complex design challenges. In contrast to traditional optimization methods, set-based design emphasizes retaining multiple feasible solutions rather than converging prematurely to a single outcome. This approach reduces the need for extensive optimization iterations, particularly in hierarchical problems, enabling a broader exploration of design possibilities while maintaining computational efficiency.
Theory
Set-based design relies on data-driven approaches to define boundaries between feasible and infeasible designs. This approach leverages machine learning (ML) techniques to classify designs based on performance criteria.
In this work, we utilized Bayesian Network Classifiers (BNC) and Kernel Density Estimation (KDE) to map the design space. These tools enabled encoding of variable dependencies and prior beliefs to more quickly converge to accurate decision boundaries. The mapping of decision boundaries is adaptable to a variety of classification techniques, offering flexibility in tackling diverse design challenges.
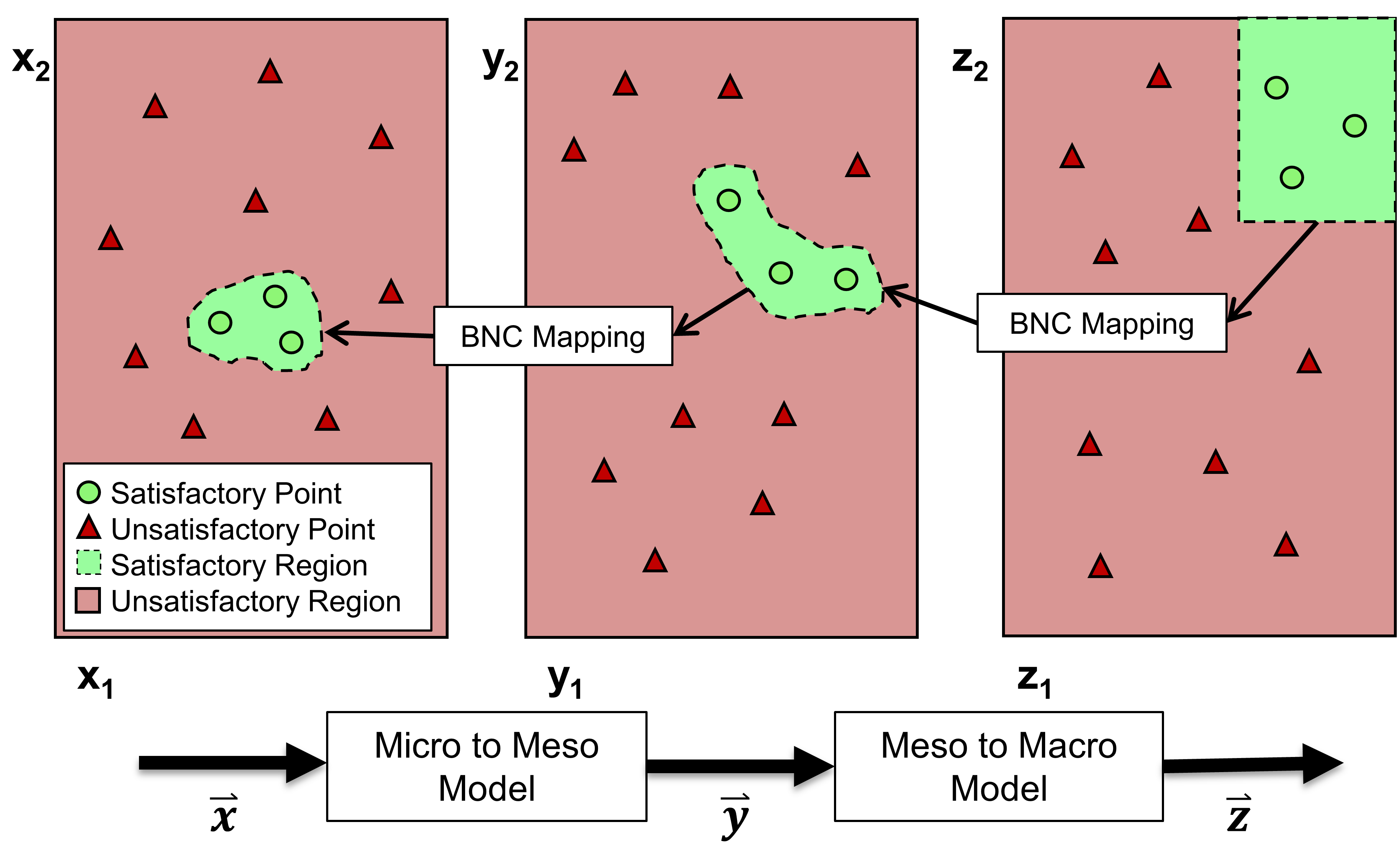
Additionally, this approach incorporates manufacturing variation directly into the design space classification process. Manufacturing variability in a part geometry can be captured using statistical distributions derived from measured data or assumed distributions. Multivariate KDE was used to model these variations, capturing both linear and nonlinear dependencies in design variables. By integrating this data into the BNC framework, decision boundaries are refined to distinguish between designs that are merely feasible and those that are robustly manufacturable.
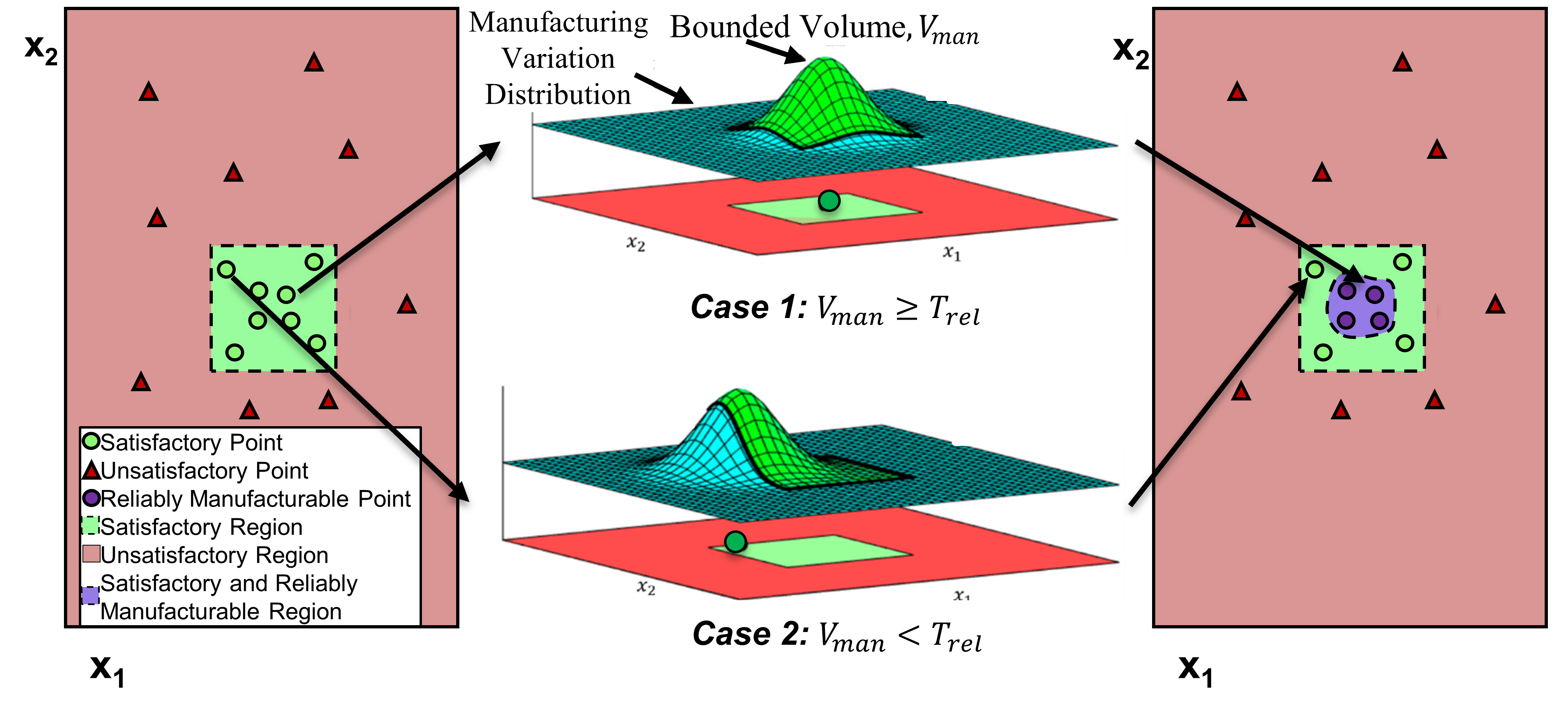
Application: Designing NS Metamaterials
The design of Negative Stiffness (NS) metamaterials is a hierarchical design problem well suited for set-based design. At the micro scale, the geometry and material properties of NS inclusions are determined. These inclusions exhibit negative stiffness behavior, amplifying strain energy for enhanced damping. At the meso scale, the host material properties and the volume fraction of inclusions dictate the composite behavior. Finally, at the macro scale, the performance criteria are enforced to select designs that are both stiff and highly damped.
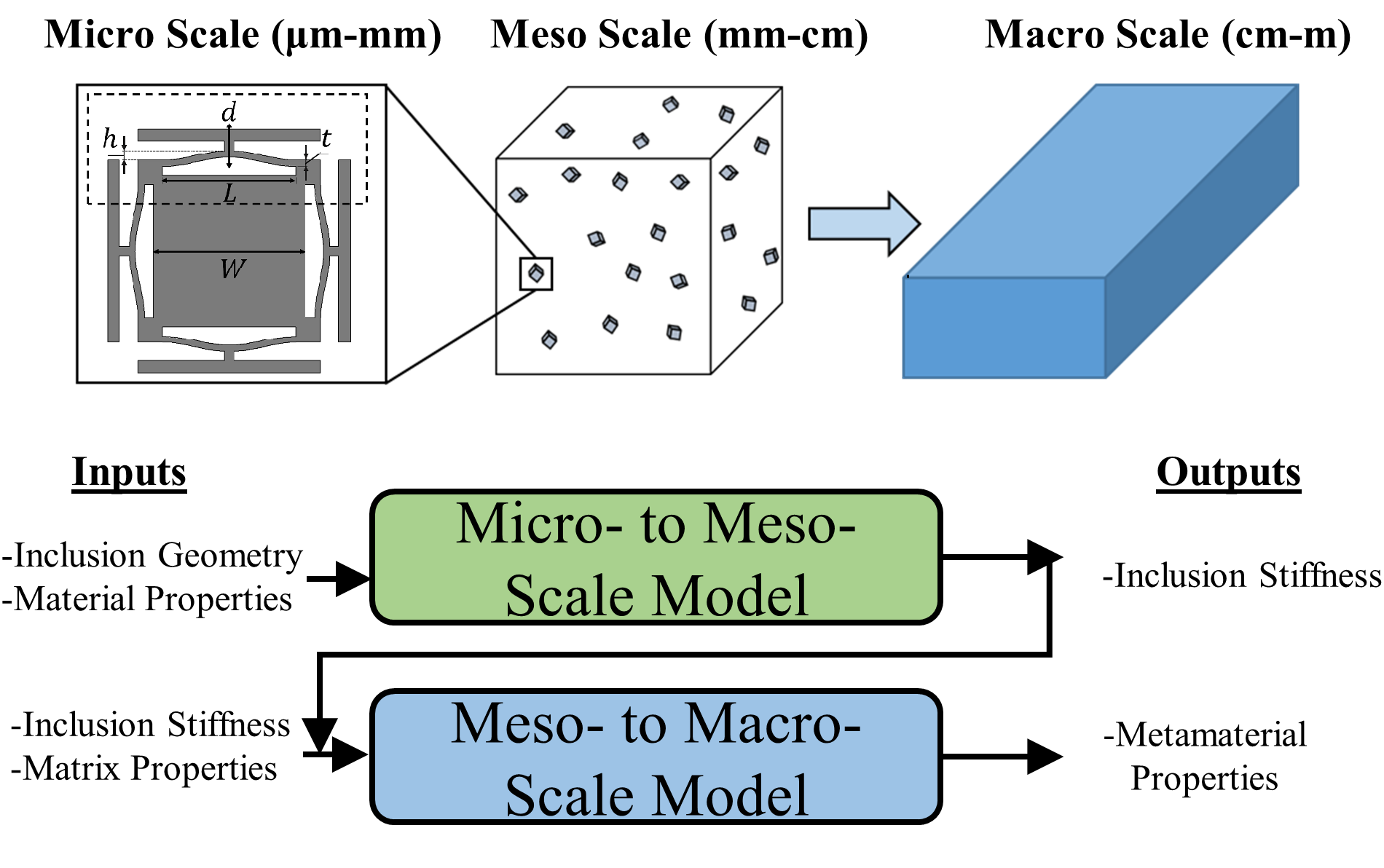
To collect the data needed to train the classifiers, finite-element analysis (FEA) is utilized at the micro-scale to determine the effective stiffness tensor of a candidate inclusion. This was achieved with a displacement-controlled approach, where the effective stiffness tensor was derived using the strain energy density. Then Effective Medium Theory (EMT) was used to obtain the complex Young's modulus of the composite material. These complex moduli were the inputs to the performance criteria where the moduli with increased damping without compromising stiffness were selected. The data at each level is then immediately labeled and used to train the classifiers.
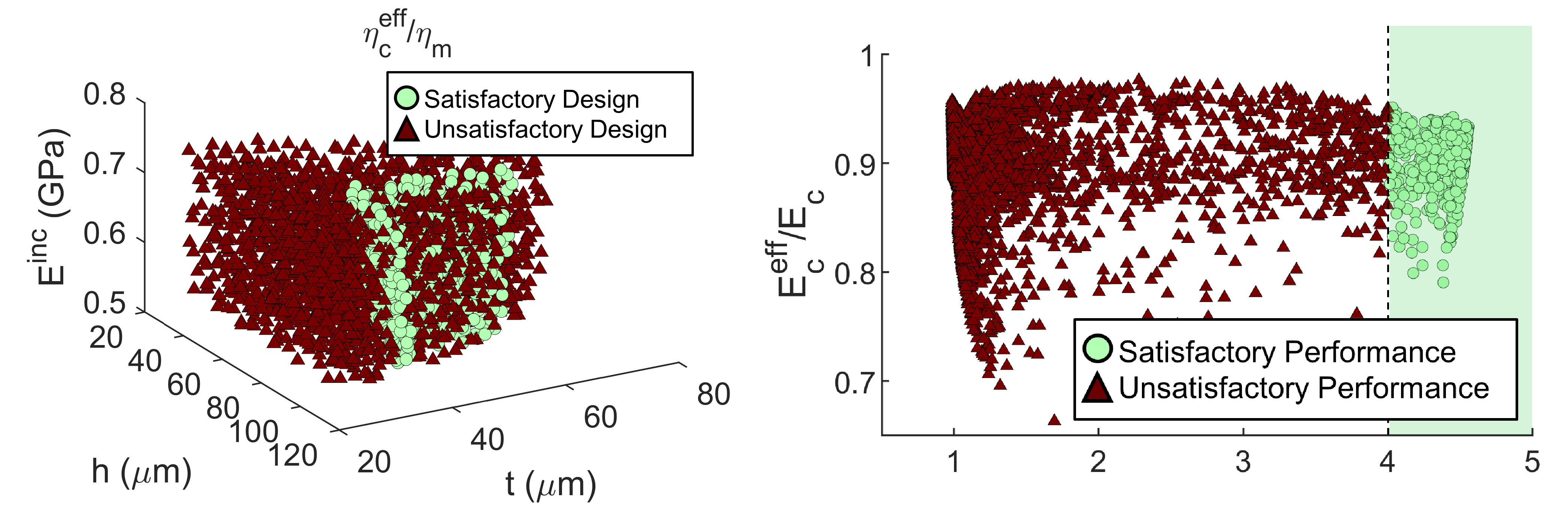
Incorporating Manufacturing Data
To incorporate manufacturing considerations, we measured beam thicknesses for a series of fabricated inclusions. These measurements yielded a statistical distribution of manufacturing variability, which was integrated into the BNC + KDE framework.
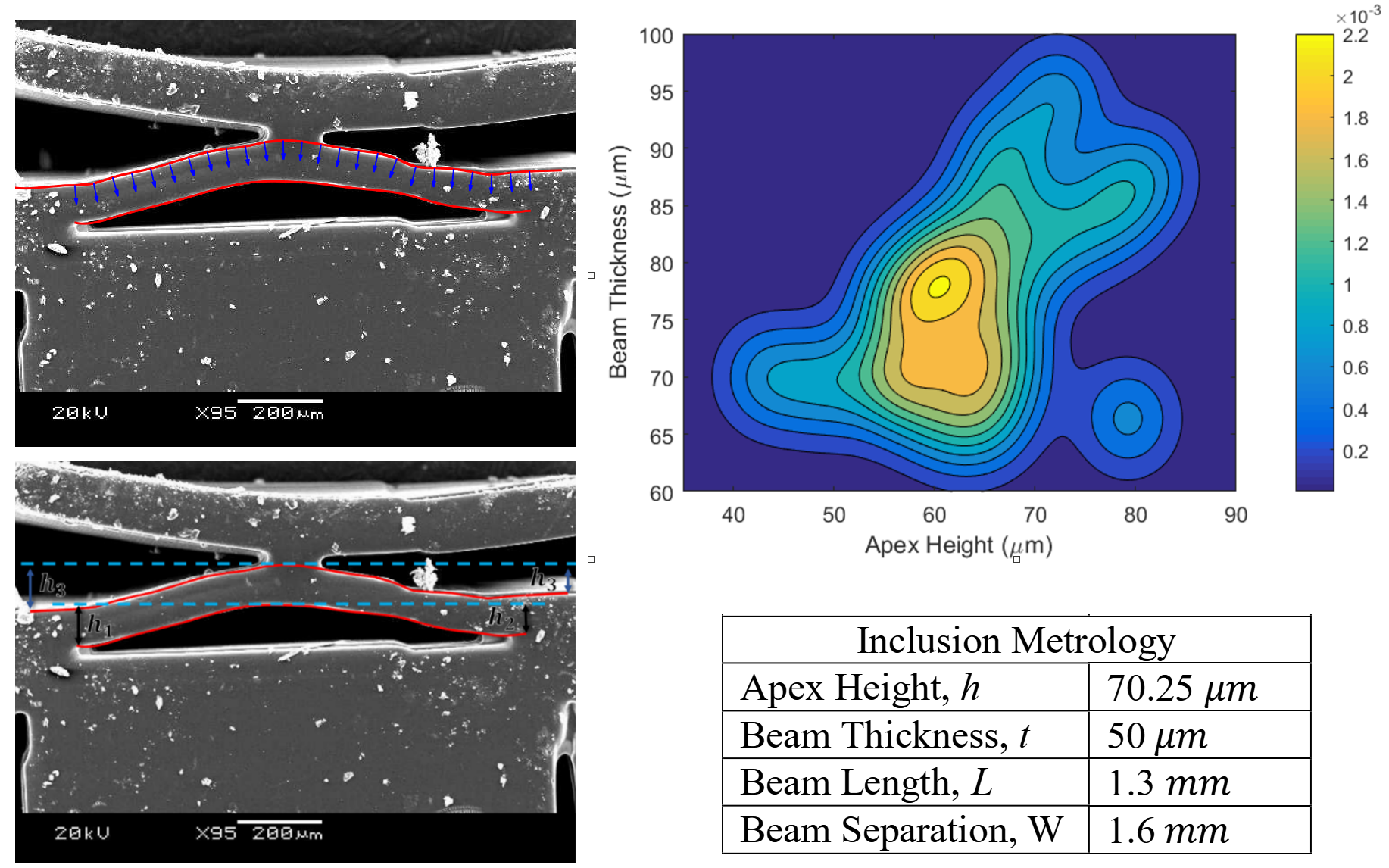
By incorporating this data, the design space was refined to identify reliably manufacturable designs. The space of feasible and robustly manufacturable inclusions was explored using a variety of reliability thresholds to illustrate the impact of reliability on the design space. After identifying robust designs, an NS metamaterial was fabricated and tested to validate the design process.
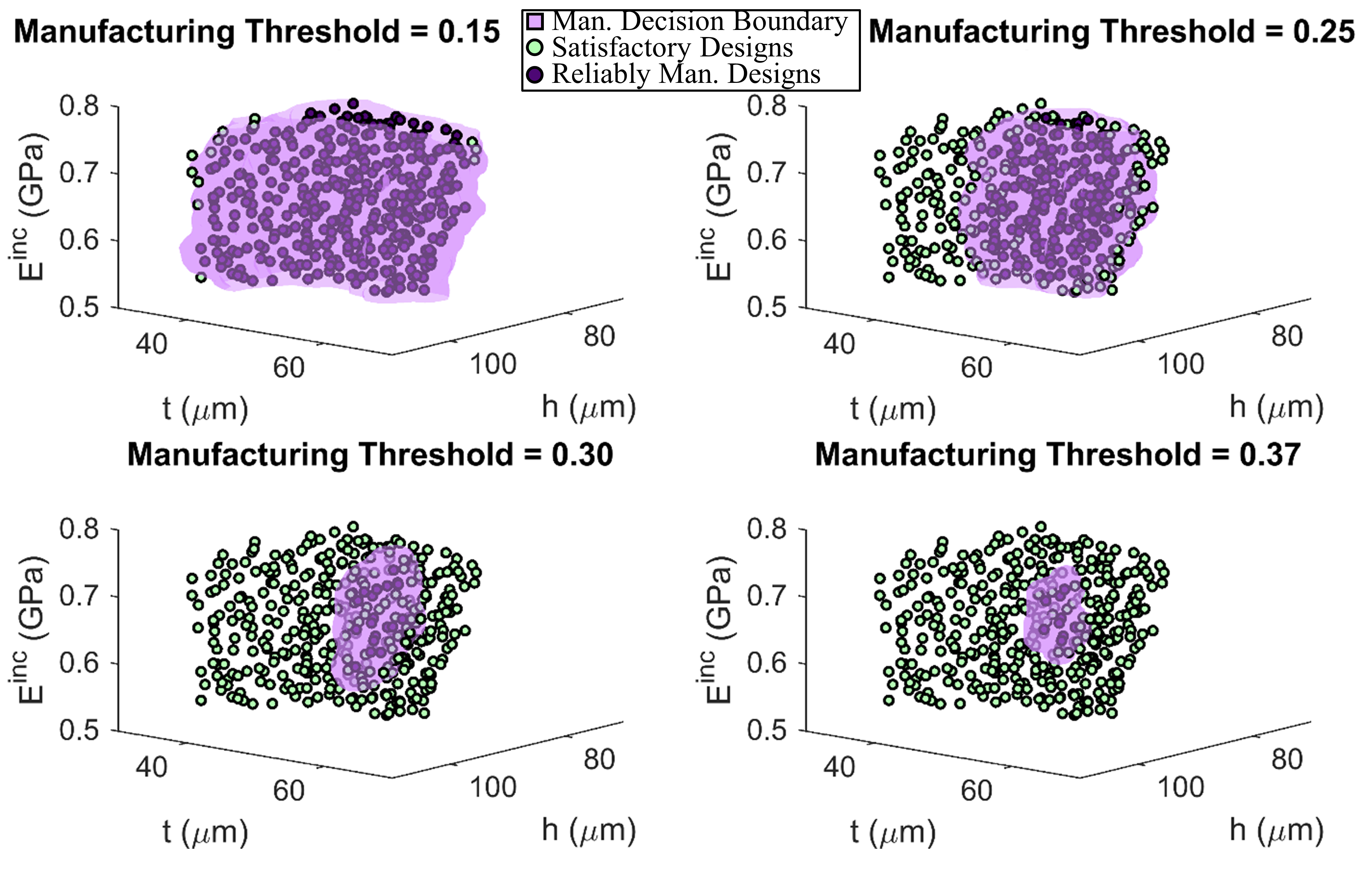
Learn More
To learn more about how these NS metamaterials were manufactured and tested, click here.
Relevant Publications
- Tunable mechanical metamaterial with constrained negative stiffness for improved quasi‐static and dynamic energy dissipation - Advanced Engineering Materials
- Design exploration of reliably manufacturable materials and structures with applications to negative stiffness metamaterials and microstereolithography - Journal of Mechanical Design
- Hierarchical design of negative stiffness metamaterials using a bayesian network classifier - Journal of Mechanical Design